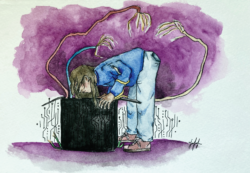
Jessie Keogh / Daily Nexus
Bias is an unavoidable part of being human. But, when bias becomes the miscalculated relic of our automated inventions, a wider problem comes into play. In an increasingly data-driven and automated world, it is no surprise that the United States has announced itself as a place of mass-market potential and efficiency.
Law enforcement relies on AI-based recommendations to identify and optimize police presence in so-called crime hotspots, like New York City and Guatemala City. Images and videos collected from AI-powered closed-circuit television (CCTV) cameras have sparked numerous debates about the ethics of privacy and mass surveillance. AI-driven fraud detectors have been deployed to recognize and block transactions that may appear illegitimate.
Decisions and judgments that have been executed by humans for decades are now shifting to automation. The seeming neutrality of identifying consistent patterns and generating data is enticing. After all, bias is the last thing we would imagine passing down to machines designed for objectivity. But, as it turns out, bias is precisely what AI has mastered.
A few computer and data scientists and advocacy watchdogs sounded an alarm in the award-winning documentary “Coded Bias.” Developing face recognition software, computer scientist and self-proclaimed “poet of code” Joy Buolamwini found that the algorithm could not detect her face until she put a white mask on. The film explores how these ubiquitous machines can not only model but perpetuate existing racial, gender and social inequalities.
In one segment, the documentary details a Houston teacher who received an arbitrarily low algorithmic evaluation despite years of awards and community recognition. Another scene uncovered the City of London Police’s use of AI-powered CCTV to racially profile pedestrians. In 2016, Microsoft unleashed the Twitter chatbox “Tay,” unaware the “robot parrot” would become a blatant racist, misogynist and anti-Semite in less than 24 hours.
An algorithmic glitch — a bad apple, some may say — has become all too common. But, what would multiple glitches or cartons of bad apples make if not a serious bout of malfunctioning?
Our blind faith in these enigmatic “black box” algorithms may only lead us to trouble. In fact, these models have encoded human bias into an apparatus that is increasingly governing our livelihoods. Truly understanding these black box algorithms is unfairly reserved for a small number of Silicon Valley tech executives and engineers, as data scientist Cathy O’Neil addressed in her 2016 book “Weapons of Math Destruction.” These “high priests’” pronouncement of what is right, wrong and objective is unquestionable. There is little room for the general public to dispute or question these systems, given that the black box algorithms remain largely opaque. And, as Buolamwini and O’Neil argue in “Coded Bias,” these heavily biased algorithms will continue to amplify the gap between the poor and rich if remained unquestioned and uncontested.
According to Kadija Ferryman, an assistant professor at the John Hopkins Bloomberg School of Public Health, “Data is never raw. It’s always cooked.” The algorithmic recipes that transform raw data into used data are encoded with deep-rooted biases that continually afflict the most vulnerable. Even with the best of intentions, humans may create AI-driven programs baked with harmful stereotypes, and therefore, these AI-driven programs have dangerous repercussions.
[Bias] is the last thing we would imagine passing down to machines designed for objectivity. But, as it turns out, bias is precisely what AI has mastered.
Take the AI-driven risk assessments, adopted in Pennsylvania in 2015, that attempt to predict recidivism, or the tendency for criminals to repeat offenses, using quantitative factors such as age, employment history, education level, socioeconomic status, neighborhood and prior criminal records. These tools help direct decisions about whether convicts will receive longer sentences, more time on parole and tougher monitoring on probation. Yet, these factors reinforce the same unjust disparities in court and society as a whole.
ProPublica performed a statistical test in 2016 in which the journalists found that 77% of Black defendants were more likely to be classified as having a higher risk of committing a future violent crime and 45% more likely to commit a future crime of any kind. Additionally, Northpointe, the for-profit company that designed the risk score algorithm in Florida, accurately predicted recidivism 61% of the time but also predicted that Black defendants were about twice as likely as whites to be classified as higher risk when they were, in fact, non-reoffenders.
In April, Silicon Valley-based AI image generator system DALL–E 2 revealed gender and racial stereotypes that overrepresented certain races and genders in certain prompts, such as generating an image of a woman for a flight attendant and a man for a builder. In January, researchers published a study that examined patient records using machine learning and found that Black patients were more than twice as likely to be described as “resistant” or “noncompliant.” Such derogatory terms may be used as “raw material” for AI programs to make predictions about patient-reported pain — oftentimes falsely classifying Black patients as having a higher pain tolerance than their white counterparts based on nothing but the notes of racially biased human doctors.
Technological neutrality is a myth. We cannot pretend that such errors are incidental. After all, the persisting biases that disproportionately affect certain demographics more than others must not be ignored – these biases directly perpetuate discriminatory stereotypes and policy decisions. While these technologies have never ceased to lend their Big Brother eye on us, it’s our turn to watch Big Tech.
In February, the Internal Revenue Service announced that taxpayers may opt out of facial recognition technology as a form of self-authentication. The third-party service, ID.me, has received significant backlash given concerns over privacy invasion. And yet, while saying a hard “no” to the growing field of artificial intelligence may be feasible in the short term, it is not the case in the unforeseeable future.
It’s becoming increasingly difficult to reverse-engineer artificial intelligence and the systems it controls. Realistically, it is near impossible to scrape clean each slowly choking tendril that AI has on society at large.
Not only that, but Big Data is ubiquitous, and advancing technologies cannot ignore that fact. An alternate approach must be taken.
Without confronting the algorithmic frameworks that perpetuate the divide among certain demographics, artificial intelligence will replicate the persisting inequalities found in our physical and cyberspaces.
In their attempt to illuminate the black box of AI, numerous data researchers and advocacy groups have endorsed algorithmic audits. While there is no federal law requiring AI systems to be regulated and audited, the importance of keeping corporate algorithms accountable is not to be disregarded. Audits can help publicly review an algorithm’s code and test out scenarios that may help gauge the efficacy and potential biases of the design. Companies must also respond to these audit reports and correct what needs to be fixed.
We must hold AI systems to a standard so that we do not blindly give faith in these algorithms nor neglect their increasing capacity and influence in society at large. When held accountable, these systems may provide us with feasible methodologies and solutions in a society governed by massive amounts of fast-moving data. Only then can we strip the opaque linings of the black box algorithms and be honest about what these technologies can and cannot do.
Melody Chen considers freedom as free as two plus two make five and that automatic machines are a hundred percent accurate …