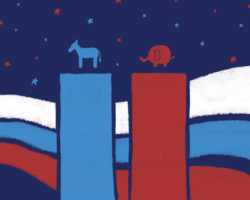
KAYLEE HEARTMAN & MICHELLE WU / DAILY NEXUS
If you flip through a few news channels in advance of the recent election, you’re likely confronted with conflicting predictions for the future leader of the United States. Professors like Allan Lichtman of American University — creator of The 13 Keys to the White House system — are gaining national news coverage for their abilities to quantify the uncertain.
UC Santa Barbara has introduced a graduate seminar on the mathematical aspects of democratic election, examining the complexities of democratic justice in a single-winner system. A research team at the University of Pennsylvania is even investigating if monkeys can predict voting behaviors. In search of an understanding over the seemingly unpredictable, Americans may turn towards the phenomena of presidential election forecasts.
Despite the overwhelming surplus of election predictions, many voters remain skeptical about whether it’s even possible to interpret the current political landscape through a scientific perspective. Thanks to three mathematical techniques and evolving AI applications, we can better interpret conflicting election projections.
The most familiar method of election prediction applies a weighted probability model. Since generating accurate election projections in the 2008 and 2012 elections, probability models have been widely broadcasted to cover federal elections. These quantitative models rely on data from survey responses, which typically includes two traditional polling questions: “How likely are you to vote in the upcoming election?” and “Who are you voting for?”
Probability models weigh data from the most accurate polls to account for known biases and form an average based on available data. Analysts then account for expected changes — a candidate gaining support in swing states, the unemployment rate increasing under the incumbent’s administration increasing voter turnout — and run many simulations under slight adjustments to generate election predictions under various likely circumstances. On Nov. 3, a probability model created by the polling aggregation website 538 cited a 52% chance of Trump’s electoral victory, meaning he won 52 times in a sample of 100 simulations.
These quantitative models can provide unreliable predictions due to polling failures. Nonresponse, dishonest partisan feedback and undecided voters in recent years have contributed to substantial polling errors which generated inaccurate predictions for the 2016 election.
To address these inaccuracies, a team of political scientists at Columbia University have developed a “fundamental-based” forecast — taking into account the complex structural factors which influence how voters cast their ballots.
Political science models consider recent changes in global affairs and the domestic economy, correlating current events with historical data to anticipate how voters will change their minds before election night. Despite producing much more stable and accurate projections in the last two election cycles, they’re criticized by statisticians for “overfitting” to historical data, meaning the equations risk collapsing when applied to predict the future .
To address weaknesses in both forecast algorithms, political analysts have begun implementing machine learning techniques. Some new prediction models go so far as to substitute polling data with AI-generated responses to instantaneously reflect the distribution of political opinions expressed online. One Harvard study conditioned a “population” of individual AI models to represent a range of viewpoints by assigning each Large Multimodal Model (LMM) different data corpus restrictions. LMMs are able to interpret data from multiple mediums or “modalities,” either written or illustrated, and apply their comprehensive understanding of social context to answer complex questions.
The researchers input different combinations of demographic features — ideology, age, gender, race — and generated AI responses to a survey questionnaire. The questions were selected from the Cooperative Election Study (CES), a digital survey of American voters to analyze trends between demographic background and political affiliation across a nationally representative sample of respondents. Within distinct ideological subgroups, the team observed a 97% correlation between CES and LMM polling results.
With any projection model, there’s a risk that viewers will confuse the probability of a candidate’s victory with their current share of votes. One study led by a Stanford researcher concluded that election forecasts often mislead the public to believe their candidates have already secured a victory, disengaging voters and decreasing votes for forecasted winners.
Though it will take time to learn if AI tools will improve forecasting accuracy, in the meantime, they raise an interesting dilemma as we risk substituting computer generated prophecies for the authentic voice of the people. Will election projections become even more disconnected from public opinion and further impact ballot boxes across the nation?
If the dead heat in presidential race predictions make you feel uneasy, remind yourself that their scientific reliability is still heavily debated by political scientists across the nation.
Electoral participation has a much greater impact on voting outcomes than any probabilistic forecast. With your strengthened understanding of the mathematical algorithms and empirical data underlying every prediction, the Nexus hopes you recognize your own role in determining the future of our democracy.
this is super interesting and well written! 💙💛
AI’s ability to forecast election outcomes keeps evolving as data models and algorithms improve. Advanced tools and platforms can process massive amounts of data for pattern recognition. Services similar to character ai customer service highlight how technology supports users with complex tasks. These forecasting methods do not guarantee perfect predictions, but they can guide analysts in interpreting polling data. The future of election forecasting may see continuous enhancements as technology grows more sophisticated.
Thanks for bringing attention to hearing issues! Here’s a resource for auditory rehabilitation: https://nychearing.com/services/auditory-rehabilitation/.