From erratic weather patterns to increasing occurrences of hurricanes, the climate crisis is worsening by the minute. The main component of climate change is greenhouse gas emissions, with methane in particular accounting for 30% of the rise in global temperature. This wide-scale emergency calls for innovative solutions that have the potential to mitigate the disastrous consequences of human activity.
Graduate student researcher Satish Kumar works for the Vision Research Lab at UC Santa Barbara and has been working on MethaneMapper, an AI-based tool that detects and quantifies levels of methane in a given area. Kumar focuses on hyperspectral imaging, a technique that analyzes a wide spectrum of light rather than assigning each pixel in an image to red, green and blue.
Greenhouse emissions are undetectable under regular vision systems, but with respect to hyperspectral imaging, Kumar said, “Since you have such a refined spectral range, you can capture any particular type of gas that you’re focusing on.” While working at the Vision Research Lab, Kumar has focused on grid detecting emissions of greenhouse gas, specifically in the setting of the oil and natural gas industry.
Coming from a background in signal processing — an engineering subfield that focuses on the analysis, modification and synthesis of signals that can be in the form of sounds, images and seismic waves. Kumar was able to incorporate this knowledge into the machine learning algorithms that make up MethaneMapper. Machine learning algorithms are designed to recognize patterns and adapt based on the data given.
“We thought we could solve the problem in a better way with machine learning compared to the existing methods which are heavily dependent on subject knowledge and are tuned [only] for certain locations. So, we thought maybe machine learning can make a network learn about everything,” Kumar said in regards to implementing machine learning algorithms.
In terms of target detection and methane identification in hyperspectral imaging, machine learning approaches are prominently used. MethaneMapper uses a hyperspectral transformer for methane plume detection. In order to improve traditional transformer designs, two modules, Spectral Feature Generator (SFG) and Query Refiner (QR) work together in order to enable the localization of potential methane hot spots in the hyperspectral images.
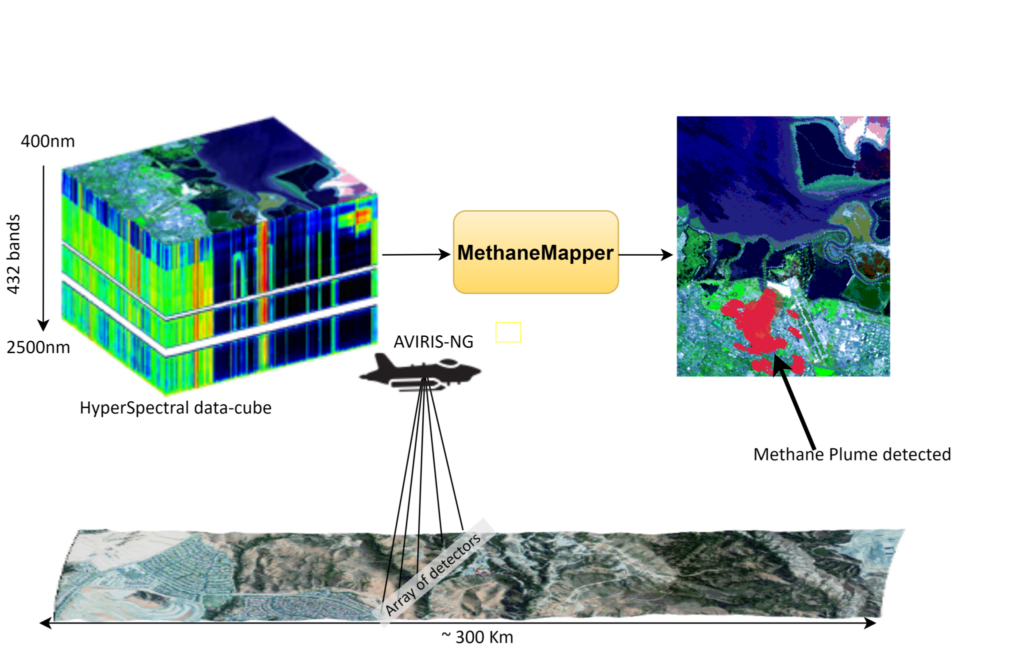
Diagram depicting MethaneMapper in action. Courtesy of Vision Research Lab, UCSB.
Both SFG and QR are machine learning systems, but the SFG takes in the channels of a hyperspectral image and processes it through a linear filter that outputs the weight mean of input values, then the QR uses the methane candidates to refine the learnable queries. In addition, the hyperspectral decoder uses the results from the QR in order to generate the final prediction of methane plumes. The system works through functional stages of data filtration, extraction, refinement, decoding and encoding.
The detection of greenhouse gasses is critical and MethaneMapper works to detect the emissions that go undetected because of the government’s struggle to find an accurate method of detection. Kumar said that in the Texas Permian Basin alone, there are 86,000 oil emissions, and like other oil extraction sites, they are emitting great amounts of methane into the atmosphere.
MethaneMapper has the potential to provide a detection system that quantifies emissions and localizes the source of emissions. It is possible that the government could implement MethaneMapper in the future as a way to quantify emissions and impose regulations on the oil and natural gas industry. Methane is one of the greatest contributors to global warming.
“The amount of damage carbon dioxide is going to have in 100 years, [is equal to the damage methane] will have in just 1.2 years. And that’s why we need to find out where the emissions are coming from, and most major emissions are from the oil and natural gas industry,” Kumar said.
MethaneMapper has been recognized as being the top 2% of all works in any given field and has been given special attention at the world’s most prestigious Computer Vision and Pattern Recognition conference, which will take place in Vancouver during late June. Kumar and his team have successfully developed a state-of-the-art AI-based tool that is highly effective at identifying methane emissions, and in doing so, has the ability to mitigate the impact of potential greenhouse gasses on the environment.
The MethaneMapper project is funded by the National Science Foundation award SI2-SSI #1664172. The project is part of “Center for Multimodal Big Data Science and Healthcare” at UCSB.
Great initiative. A practical and innovative tool to measure methane emission. I hope governments start using it as early as possible and fight climate change.
I made over $700 per day using my mobile in part time. I recently got my 5th paycheck of $19632 and all i was doing is to copy and paste work online. this home work makes me able to generate more cash daily easily simple to do work and regular income from this are just superb. Here what i am doing.
.
.
.
For Details►—————————➤ https://EarningDoors1.blogspot.Com
Katharine Chi tells us:
“…the climate crisis is worsening by the minute.
…
“The amount of damage carbon dioxide is going to have in 100 years, [is equal to the damage methane] will have in just 1.2 years.”
Why not just say how much global warming methane will cause in 100 years, and if she claims it’s more than 0.1°C she should show her work or source.