In science within academia, which has historically been dominated by white men, the issue of bias has long posed an obstacle to objectivity in fields intending to engage with and answer questions about human beings, such as anthropology, medicine and psychological sciences.
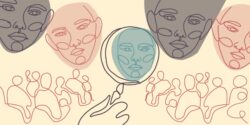
Daniela Gomez / Daily Nexus
Even the most innocent and well-intentioned of people fall prey to their own unconscious bias. The nature of human sociality makes it incredibly tempting to place greater stock in those similar to you — often much to the detriment or neglect of others.
Take in-group and out-group bias: Young children themselves have been seen withholding help from those who victimize people in their in-group, while assisting with little hesitation those who wreak havoc on people outside of this in-group.
More broadly, the observed tendency toddlers have to preferentially help those in one’s in-group when resources are scarce serves as another embodiment of bias.
One broader and structural example of bias in academia is seen in the oversampling of American college students — who tend to be white — in psychological studies. This was brought to light and acknowledged as an issue in 2010, more than a decade ago. Then, anthropologist Joe Henrich and psychologists Steven Heine and Ara Norenzayan, researchers with the University of British Columbia, found that undergraduate students from Western, educated, industrialized, rich and democratic (W.E.I.R.D.) societies comprised as much as 80% of participants in studies while making up only 12% of the world population.
But there are more concerns with the fallibility of data collection methods than just within the realm of academics. When data itself is skewed, how do the findings themselves — or even policies and outcomes — get affected by such things?
In the era of big data, when such information is increasingly of consequence to our daily lives, these questions reign supreme. As data and artificial intelligence is used more and more to delegate decision-making to algorithms and make judgment calls once taken upon by human beings — who we’d be remiss not to acknowledge came with their own prejudices and bias — concerns over how this disproportionately affects minorities, and particularly Black people, are raised with more and more frequency.
Researchers have shown, for instance, that algorithms intended to correct for human bias in dispensing things like loans and mortgages in the U.S. credit market still did very little to close the gap in default rates between white people and Black and Hispanic borrowers.
The fields of medicine and criminology are also often problematic in this regard.
Data linkage in healthcare, for instance, has been found to decrease quality of care among minorities in general. Linkage, the process in which data from providers is kept for use in monitoring, planning, research, policy development and other evaluations, is more likely to contain incorrect data or even lack important data among ethnic minorities. This can be due to things like different naming conventions, a not-unfounded lack of trust in health systems and how data is used and a tendency for minorities to frequent health facilities with relatively poor data quality.
Among Black Americans specifically, an algorithm managing care for 200 million in the U.S. annually has recently come under scrutiny for its tendency to systematically discriminate. According to a study led by a researcher with the UC Berkeley School of Public Health, “at a given risk score, Black patients are considerably sicker than white patients, as evidenced by signs of uncontrolled illnesses.”
In other words, in a scenario wherein Black people and white people were equally sick, the algorithm was significantly less likely to refer Black people to programs intended to improve their care. Such a disparity is especially egregious since the advent of the COVID-19 pandemic, wherein Black Americans were 2.5 times more likely to be hospitalized from COVID and 1.7 times more likely to die from it.
Similarly, the already incredibly controversial practice of predictive policing has come under fire from many for simply perpetuating racist policing practice. This is by virtue of the fact that “in numerous jurisdictions, these systems are built on data produced during documented periods of flawed, racially biased, and sometimes unlawful practices and policies,” according to researchers with New York University and Northeastern University.
On campus, many researchers have sought to level this uneven playing field and have taken steps to correct these inequities and issues inherent in data, for instance, by attempting to bring in more Black students to fields like data science and other disciplines in S.T.E.M. Initiatives championed by CBSR, the Center for Black Studies Research at UC Santa Barbara, like Student Engagement and Enrichment in Data Science, or S.E.E.D.S., aim to establish a “new and student-driven foundation through a data science living and learning community for understanding issues of personal significance through a diverse lens.”
Meanwhile, cross-cultural research has exploded in large part to address the issues which have arisen from Western academia’s preoccupation with W.E.I.R.D. students. Those in the anthropological sciences have sought to collaborate with others from across the world hoping to gain insight in how circumstance and other contextual characteristics impact human beings and their behavior, whether one is comparing socioeconomic status, geographic location or any number of qualities which impact why people do what they do and act as they do.