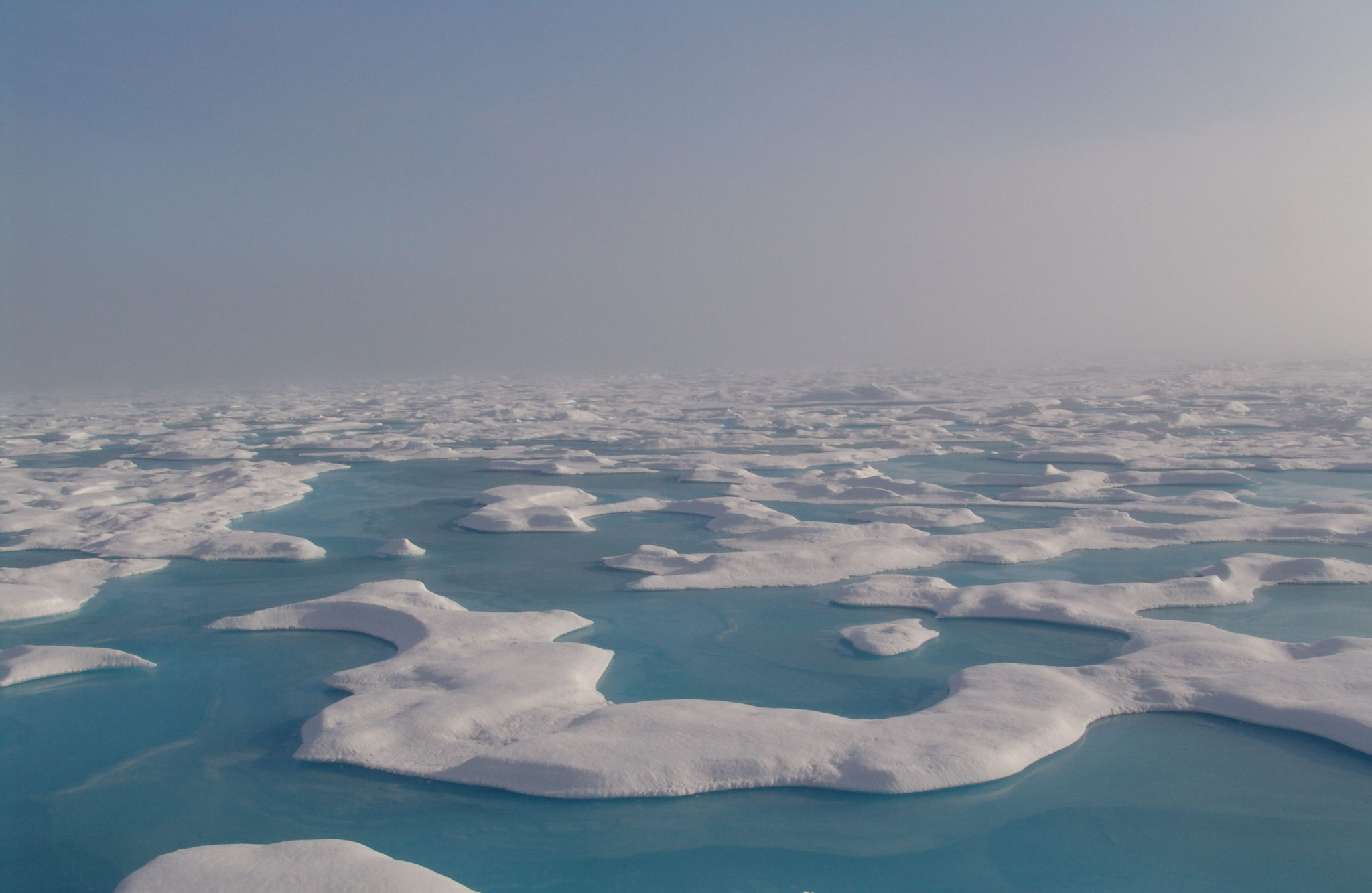
With a grant from the National Oceanic and Atmospheric Association, Ding will evaluate climate models of Arctic sea ice to better determine how to optimize their efficiency. / Courtesy of Wikimedia Commons
A professor in UC Santa Barbara’s Earth Research Institute, Qinghua Ding, has received a $200,000 grant from the National Oceanic and Atmospheric Association Climate Program Office in the Modeling, Analysis, Predictions and Projections Program for his proposal to evaluate climate models and understand what makes some more accurate than others and why.
With the newfound funding, Ding intends to judge climate models along a newly established rubric, ranking their methodologies and providing input with regard to how models can better reflect reality and improve the accuracy of their predictions.
“We’ll start from observation. We have a benchmark. Do we know this from observation? Because we only have 40 years [of] data to work with. Based on this, we decide on metrics then we use these metrics to put on the model and apply to the model something akin to a correlation,” Ding, an assistant professor in the Department of Geography, said.
With a focus on Arctic sea ice, Ding will cross-reference many different models in order to better understand how to optimize their effectiveness and which models at present are the most efficacious in predicting conditions.
“The main goal is to say which model will do the best job for Arctic sea ice simulation. We’ve tried to design some metrics and we’ll use these metrics, apply the same metrics to a model and then we’ll cast ranking based on these criteria,” Ding explained.
Climate models must take into account not only the breadth of variables affecting conditions but also the degree to which a variable is responsible for altering the state of things. There are many different attributes to take into account.
Determining the strength of the relationship between a given driver of melting and the corresponding response is key. According to Ding, many models fail because, although they include all of the measurable impacts, they do not properly gauge the significance of variables relative to one another.
“There are many challenges which arise in building long-term climate models, especially with regards to sensitivity,” Ding said.
Accuracy becomes more challenging as more variables are put in. Putting in more components to the model is necessary to best reflect reality but concurrently as more components are introduced, forecasting an outcome is difficult due to the uncertainty.
“The climate model includes a lot of chaotic features. It’s active internal variability, generated by the model itself to take into account natural variability, because so many components can determine the outcomes,” Ding stated.
Sea ice, for example, can be influenced by a variety of different factors. Sea ice touching land will melt more due to conduction, the thickness of the sea ice will influence the rate of melting, and strong winds can blow sea ice into the North Atlantic and in turn increase the rapidity of melting. Due to this, measuring net impact can be very difficult.
“So many factors can influence and have some impact on sea ice…and the modeler, the model developing group, they have no idea which is more important,” Ding said.
“It’s a really complex model. It’s a pretty well-developed code. The problem is that most people have no idea how to make the model behave in a balanced way. We don’t know which way is the right way,” he continued.
With such a large amount of things to model and different variables to take into account, sensitivity is key and also, unfortunately, the measure which is perhaps the most difficult to perfect.
Ding and his research group will collaborate with the National Oceanic and Atmospheric Association and the Geophysical Fluid Dynamics Laboratory at Princeton University. They will also collaborate with NASA and employ their exhaustive datasets.
Ding plans to complete the assessments within the next two to three years. Two undergraduate students will work alongside him.
“I hope after the project we can give some suggestions for the model developer about how to improve their model further. We don’t want to just see a model as ‘bad’ or ‘worse’ or as ‘good.’ We want to tell people how to improve their model and correct imperfections.”